Augmenting physics simulators with neural networks for model learning and control
Author(s)
Ajay, Anurag.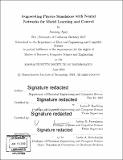
Download1124767014-MIT.pdf (7.284Mb)
Other Contributors
Massachusetts Institute of Technology. Department of Electrical Engineering and Computer Science.
Advisor
Leslie P. Kaelbling and Joshua B. Tenenbaum.
Terms of use
Metadata
Show full item recordAbstract
Physics simulators play an important role in robot state estimation, planning and control; however, many real-world control problems involve complex contact dynamics that cannot be characterized analytically. Therefore, most physics simulators employ approximations that lead to a loss in precision. We propose a hybrid dynamics model, combining a deterministic physical simulator with a stochastic neural network for dynamics modeling as it provides us with expressiveness, efficiency, and generalizability simultaneously. To demonstrate this, we compare our hybrid model to both purely analytical models and purely learned models. We then show that our model is able to characterize the complex distribution of object trajectories and compare it with existing methods. We further build in object based representation into the neural network so that our hybrid model can generalize across number of objects. Finally, we use our hybrid model to complete complex control tasks in simulation and on a real robot and show that our model generalizes to novel environments with varying object shapes and materials.
Description
Thesis: S.M., Massachusetts Institute of Technology, Department of Electrical Engineering and Computer Science, 2019 Cataloged from PDF version of thesis. Includes bibliographical references (pages 77-81).
Date issued
2019Department
Massachusetts Institute of Technology. Department of Electrical Engineering and Computer SciencePublisher
Massachusetts Institute of Technology
Keywords
Electrical Engineering and Computer Science.